What is the Rag? Role about RAG and Generative AI
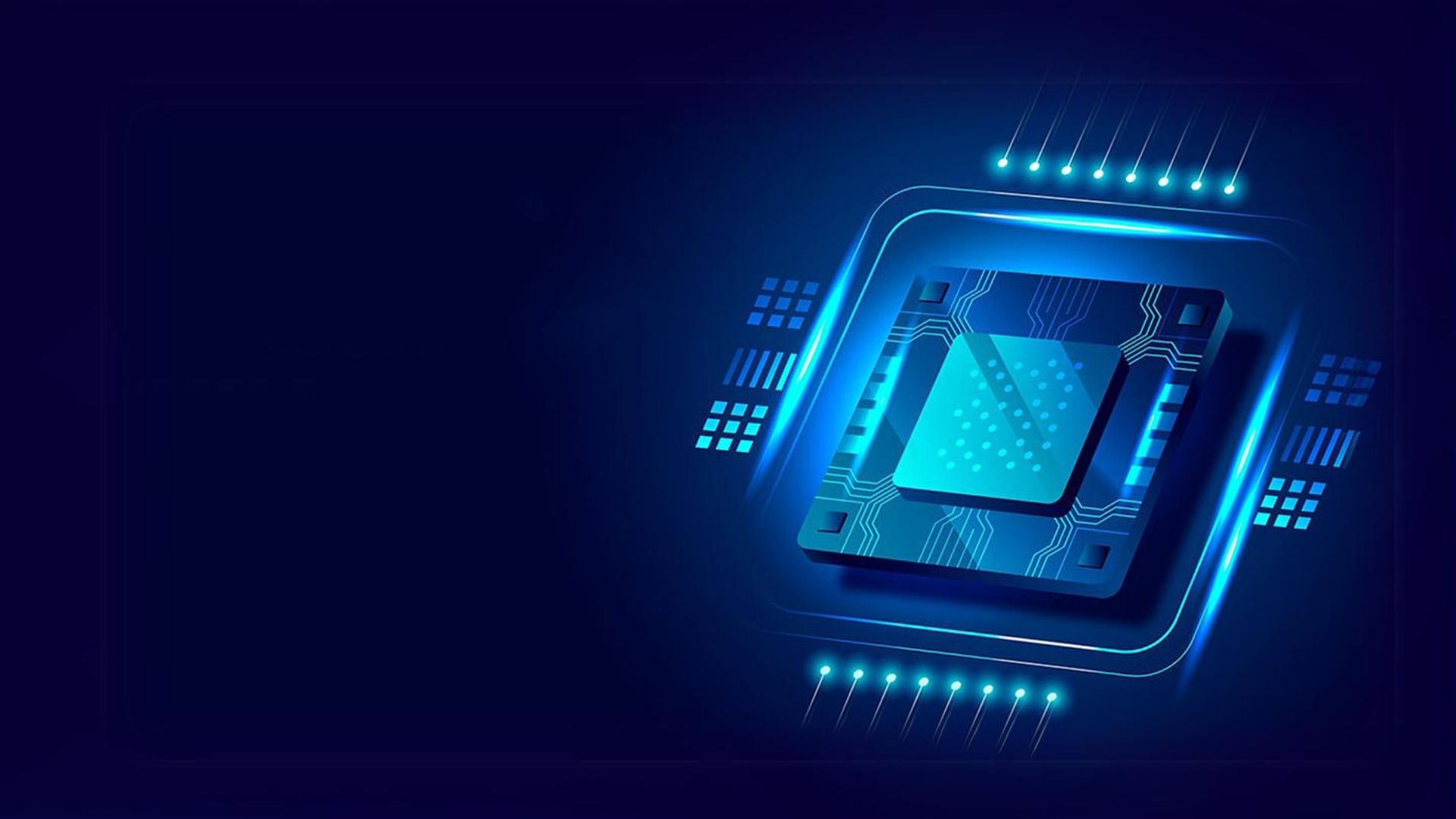
As AI technology is a part of today’s Rag ai use cases in everyday life. It continues to reshape industries; businesses are looking for ways to enhance the performance of their AI systems while keeping costs low. One of the most promising developments is Retrieval-Augmented Generation (RAG), which supercharges large language models (LLMs) by integrating them with external data sources. By using RAG, companies can optimize their AI outputs with current, domain-specific information, making responses more accurate and reliable. This advancement is especially crucial for businesses seeking to implement generative AI in fields that require timely and trustworthy data.
What is RAG? Role of RAG in Generative AI
Retrieval-Augmented Generation (RAG) is a method that enhances the output of large language models (LLMs) by allowing them to reference external data sources before generating a response. Traditionally, LLMs are trained on vast datasets but remain static and limited in knowledge over time. RAG extends the LLM's capabilities by enabling it to retrieve and incorporate information from updated, authoritative sources. This ensures that the AI provides more accurate, relevant, and timely responses without needing extensive retraining. Businesses, especially in fast-paced industries, find RAG particularly beneficial for maintaining current and high-quality outputs from AI systems.
Why RAG Matters in Generative AI?
Retrieval-Augmented Generation (RAG) plays a pivotal role in enhancing the functionality of Large Language Models (LLMs), which excel in natural language tasks but struggle when limited by outdated or insufficient training data. RAG addresses this gap by enabling LLMs to retrieve and integrate real-time information, ensuring responses are relevant and accurate. Additionally, RAG fosters trust by providing source citations and allows developers to fine-tune model output without needing costly retraining. This makes it an appealing, cost-effective solution for businesses leveraging generative AI.
Key Advantages of RAG in Generative AI
1. Ensuring Relevance and Accuracy
RAG helps models provide up-to-date responses by fetching real-time data from external sources. This resolves common issues in LLMs where responses become outdated or miss the specific context needed for certain queries. With RAG, businesses can confidently rely on AI outputs that provide Quality Lead Generation, whether it's for customer service, market research, or internal decision-making.
2. Enhancing Trust through Source Attribution
Another advantage of RAG AI use cases is its ability to cite the sources of the information it retrieves, offering transparency in AI-generated responses. This boosts user confidence in the technology, as they can verify the accuracy of the AI’s output themselves. This attribution system is particularly important for industries like healthcare, law, or finance, where precision and accountability are crucial.
3. Greater Developer Control
RAG provides developers with more control over their AI systems by allowing them to customize the external data sources that the AI references. This enables developers to fine-tune the LLM’s performance for domain-specific needs or adjust the output based on changing requirements. This flexibility gives businesses the ability to deploy AI solutions across various functions without losing control over the quality of the responses.
4. Cost-Effective Solution
Compared to retraining a model from scratch, RAG is far more economical. Traditional AI retraining processes are resource-intensive, often requiring vast amounts of new data and computational power. RAG bypasses this by simply integrating new information in real time. As a result, businesses can continually improve their AI optimized content strategy without incurring the high costs associated with retraining, making RAG an ideal approach for companies looking to scale AI solutions affordably.
What are the benefits of RAG?
The benefits of Retrieval-Augmented Generation extend beyond improving AI output. From cost savings to increasing user trust, RAG provides several advantages that make AI systems more practical and powerful. For organizations looking to deploy generative AI solutions, these benefits ensure that their systems are not only efficient but also reliable and adaptable to various industry needs.
If you want to know if we can help your business with RAG, then a detailed discussion is recommended. Get in touch with out team.
How RAG Works
RAG optimizes the response-generation process by augmenting LLMs with real-time data retrieval. Here’s a closer look at how this works:
· Creating External Data:
External data is converted into numerical vectors that are stored in a vector database. This could be information from APIs, document repositories, or other data sources outside the LLM's original training set.
· Retrieving Relevant Information:
When a user query is received, it’s converted into a vector format and matched with relevant data from the vector database. This allows for more precise information retrieval, ensuring that the AI provides a detailed and accurate response.
· Augmenting the LLM Prompt:
Once the relevant data is retrieved, it’s added to the LLM’s prompt. This process helps the AI create responses that combine pre-trained knowledge with up-to-date, domain-specific information.
· Maintaining Data Relevance:
To ensure that the AI's outputs remain current, external data is periodically updated or refreshed. This optimized content strategy process can be automated, ensuring that the AI’s responses are always based on the most recent information available.
Leadmetrics AI and RAG Integration: Enhancing Lead Generation
Leadmetrics AI combines the power of RAG with its lead generation software to provide businesses with an advanced, data-driven approach to capturing and converting leads:
· Contextualized Data Retrieval:
By pulling in data relevant to the user’s industry, Leadmetrics ensures that the AI provides accurate lead-generation insights. This allows businesses to better understand potential customers and market trends.
· Real-Time Market Updates:
Leadmetrics integrates live data sources, including social media feeds and market news, into its system, enabling the AI to generate more relevant and timely insights for lead nurturing.
· Enhanced Personalization:
By utilizing external data, Leadmetrics AI tailors its lead-generation strategies based on the most current trends, allowing businesses to create personalized marketing campaigns that resonate more effectively with potential clients.
· Data-Driven Lead Scoring:
The software leverages RAG to provide enhanced lead scoring capabilities. By using both historical and real-time data, it accurately ranks Quality Lead Generation, helping businesses prioritize high-value opportunities.
· Optimized Follow-Ups:
Leadmetrics AI ensures that businesses can follow up with leads at the right time, using the latest data to inform engagement strategies and maximize conversion rates.
Frequently Asked Questions
What is Retrieval-Augmented Generation (RAG)?

Retrieval-Augmented Generation (RAG) is a method that enhances large language models (LLMs) by allowing them to reference external data sources before generating responses, ensuring that the information provided is more accurate, relevant, and timely.
Why is RAG important for generative AI?

RAG is important because it addresses the limitations of traditional LLMs, which can become outdated. It allows them to access real-time information, improves response accuracy, fosters user trust through source citations, and reduces the costs and resources required for retraining.
How does RAG improve accuracy and relevance in AI outputs?

RAG improves accuracy and relevance by fetching real-time data from external sources when generating responses. This allows businesses to rely on up-to-date information, helping them in areas like customer service, market research, and internal decision-making.
What are the main advantages of using RAG for businesses?

The main advantages of RAG for businesses include ensuring the relevance of AI outputs, enhancing trust through source attribution, providing greater control to developers over data sources, and offering a cost-effective solution compared to traditional AI retraining.
How does RAG work in practice?

In practice, RAG works by converting external data into numerical vectors stored in a database. When a user query is received, the system retrieves relevant data, augments the LLM's prompt with this information, and generates responses that are current and contextually relevant.
Share on Social Media
Ready to start
sourcing leads Online?
Explore more with Leadmetrics